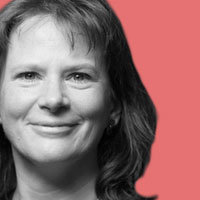
Increasingly, we see examples of advanced technologies deemed to be out of step with social norms and requirements such as privacy, transparency, accountability. 2019 saw the State rebuked for the misuse of data via the Public Services Card, Facebook removing ‘coordinated inauthentic behaviour’ from its site, and Amazon discontinuing use of a recruiting tool found to be biased towards male candidates. This article outlines measures that should be taken to minimise risks and enhance the positive contribution of AI to the digital society.
Artificial intelligence applications promise to be some of the most powerful knowledge technologies ever developed, supplementing and shaping how we develop our understanding of the world, as they will not only supplement human capacities but in many cases outstrip them. Given the potentially very sharp double edge on this sword, what measures should we be taking to minimise any risks and enhance AI’s positive contributions to the digital society?
The vision behind the term ‘digital society’ is that digital technologies maximally support the functions of society to govern, educate, heal, connect, and protect its members. Ireland launched its first National Digital Strategy in 2013 (Department of Communications, Energy and Natural Resources, 2013), with the goal to be ‘a foundation step in helping Ireland to reap the full rewards of a digitally enabled society’. To read this optimistic document now is to be reminded of how far our awareness has come of the potential effects of technology on our societies and our lives since that time, when the greatest concern seemed to be that Ireland might miss the wave of prosperity that ‘doing more with digital’ could bring us.
A public consultation to revise the strategy and extend it beyond phase one status was launched in October 2018. Over 300 responses were received, as yet unpublished, and in May 2019 the Taoiseach confirmed that the new strategy is under development, overseen by an interdepartmental committee. In July 2018, the government released a report in partnership with the Microsoft Applied Innovation Team and the Fletcher School at Tufts University. Entitled ‘Enabling Digital Ireland’ (Office of the Chief Information Officer, Dept. of Public Expenditure and Reform, and Microsoft Ireland, 2018), it portrays the role of digital in improving society very differently from the 2013 document, positioning itself far more as an e-government strategy than a comprehensive digital society agenda.
We can see similar trends at European level, with widely varied digital society visions and policies being underpinned by sweeping narratives of progress through technological adoption. But in spite of lofty rhetoric in reports such as 2019’s ‘Digital Europe’ on how the digital transformation touches ‘every aspect of our lives’ (European Commission, n.d.) the only perspectives really represented in this case are those of software developers and researchers, according to which we have not ‘collectively invested enough in the latest technologies’. The technological imperative makes reference to and seems to assume a basis in an underlying social good, but this is far from proven.
Whether we take a narrow or a broad perspective on how technology should support society makes a big difference to how we view the best modes for introducing new technologies like AI. Defining social problems as engineering challenges can often lead to blind spots and unconscious biases entering the system. Similarly, if we view the digital society too narrowly and optimise only one aspect of it, like efficiency of services, other necessary aspects, like privacy, can be quickly and gravely compromised.
Even before we had computers, we had data and statistics. Ledger books tracking trade flows and legal transactions can be found as far back as the material record takes us, and using mathematical and operational models to track such flows is an innovation of the second industrial revolution, not the fourth. Yet the difference in scale, speed, and complexity of the models and the material they are trained on makes the current moment and the potential it holds seem a difference in kind, not just degree.
AI has a variety of meanings, representing both software systems and the scientific disciplines that create them. The European Commission’s High-Level Expert Group on Artificial Intelligence therefore began its work by defining its object of study:
Artificial intelligence (AI) systems are software (and possibly also hardware) systems designed by humans that, given a complex goal, act in the physical or digital dimension by perceiving their environment through data acquisition, interpreting the collected structured or unstructured data, reasoning on the knowledge, or processing the information, derived from this data and deciding the best action(s) to take to achieve the given goal. … As a scientific discipline, AI includes several approaches and techniques, such as machine learning (of which deep learning and reinforcement learning are specific examples), machine reasoning (which includes planning, scheduling, knowledge representation and reasoning, search, and optimization), and robotics. (HLEG on AI, 2019a)
These definitions remind us of the breadth of activities included under AI. They also remind us of how different AI as we now know it is from how science fiction has taught us to imagine it. From the uncanny animated mechanical dolls found in nineteenth-century literature, to the modern-day disembodied AIs such as Samantha (from the 2013 film Her), our imaginations tend to start from the human and add or subtract attributes to imagine the machine.
This tendency encourages us to think in terms of what is called general (or strong) AI rather than narrow (or weak) AI. In spite of the amazing progress made in developing software that can achieve human-like, even superhuman, results in such human activities as playing chess or Go, general AI is still very much in the future. In spite of what it may seem like a computer can do, even the most human-like communication abilities of software like Google’s Duplex project are narrow in their application (in this case making restaurant or salon reservations convincingly).
To understand the potential of AI for both good and ill, therefore, we must divest ourselves of the robots and sentient software of our imagination, and focus instead on the idea of systems that can perceive or sense, reason, learn, and or make decisions, and finally invoke an action based upon these processes. No doubt this is an exceptionally powerful set of capacities, but it falls far short of true human flexibility and capability. The responsibility remains with us humans to decide how we might create and use software agents with these capabilities as a positive supplement to existing human social interactions and processes.
Recognising the potentially broad, but not necessarily always positive, impact of AI on society, the Expert Group mentioned above also developed Ethics Guidelines for Trustworthy Artificial Intelligence (HLEG on AI, 2019b). The guidelines call for AI to be lawful, ethical, and robust, but they also put forward seven key requirements that AI systems should meet in order to be deemed trustworthy. These stand as a reminder of exactly how AI implementation might go wrong, calling for:
The modelling of social processes that lies at the heart of the actions that AI will effect is always based on an incomplete view of the complexity of human motivations and interactions. As the saying goes, all models are wrong, but some models are useful. How will we know the difference, or at least the limits?
Most pressingly, as these technologies become ever more mature and widespread, how will we know what social processes we can and cannot turn over to systems based on AI? Already we have seen the kinds of human costs paid from this impetus. Uber drivers report that their management by machines is detrimental to their sense of job satisfaction and well-being (Möhlmann and Henfridsson, 2019). Others have been denied job interviews, insurance, and even parole based in part on the conclusions of software algorithms that may have been inappropriately biased against them (O’Neil, 2016).
Although there are clauses in the European General Data Protection Regulation (GDPR) that give EU citizens the right to know the basis for any algorithm-based decisions made against them, there may be as much reason for concern about advanced knowledge technologies that work well as about those that result in obvious unintended negative consequences. The mass adoption of social media platforms, for example, speaks volumes about how well they respond to the social and psychological requirements of their many users. But they have also become agents through which our capacity for tolerating different viewpoints has been diminished, where the spread of hate speech and purposeful disinformation has been facilitated to the detriment of democracy, and such fundamentally human resources as our attention and our privacy have become monetised and sold, largely without our awareness or any real form of consent.
The arts and humanities, with their traditions of critical thinking, cross-cultural understanding, emphasis on knowledge creation via empathy, and approach to challenging ethical and moral questions, develop many of the skills we will need to ensure that advanced technologies such as those based on AI are adopted in ways that support social justice and community cohesion. In addition, these disciplines deal with complex human problems that are not reducible to discrete parts or controlled experiments.
In this case, AI will increase rather than decrease the requirement for problem-solving, as even the engineers building this software may not understand all the nuances of the mechanisms by which the machine-learning processes reach the conclusions they do. The competence to weigh evidence and reject easy conclusions will be an ever more essential counterweight to foreign governments and companies that may want to unleash (or not see the harm in unleashing) software that disrupts public discourse and democratic processes.
The future will require us to grow the number of citizens who understand the workings and limitations of the AI that will inevitably come to underpin our social processes. Indeed, the Finnish government’s release of a free and open online course (University of Helsinki and Reaktor, n.d.) that explains, in simple and straightforward language, the underlying techniques and assumptions of AI is an inspiring example of how this awareness might be delivered.
But more than anything else, AI’s capacity to make social processes reliant upon technological black boxes will require societies to make a commitment to shared values and goals before these systems are deployed. This very analogue process of explicit dialogue, education, and perhaps even active resistance to the worst inclinations of a profit-driven system will be one of the most important enablers for the digital society.
Making citizens aware of how AI may be shaping their social and individual lives, and empowering them to engage wisely with it, is one way in which the promise of the digital society can be assured. This cannot be the only way, however. Placing responsibility for the unintended consequences of a profitable industry on the consumers of that industry’s products is at best naïve and at worst a convenient and egregious form of victim-shaming. Technologies that are destined to become part of our digital society must therefore also be regulated from the top down, just as users are educated from the bottom up.
If we are looking for models by which to structure an approach to regulating knowledge technologies based on big data and AI, there is perhaps no better place to look for inspiration than another industry that revolves around things that can heal or harm: pharmaceuticals.
First of all, drugs, like technology, emerge from a sophisticated research pipeline, but still need to be rigorously tested to tease out any possible unintended consequences or side effects of their widespread use before they can be sold. Even after being approved, their use may be subject to restrictions, and they will probably not be available without the case-by-case approval of a medical professional, the cornerstone of whose education is an oath to ‘first, do no harm’. These professionals are also required to abide by a professional code of conduct to maintain their right to practise and to put the health of their patients above personal profit motives.
While the regulatory system for pharmaceuticals is by no means perfect, it provides a set of possibilities to consider for how other research outputs might be meaningfully regulated. In the final analysis, to know how best to deploy AI and the data that feeds it for the good of the digital society will require governments, communities, and individuals alike to consider carefully what they want their lives and communities to look like. The hardest part of building AI will most certainly be creating a consensus about what we want it to do.
Copenhagen Letter (2017) https://copenhagenletter.org/.
Campaign to Stop Killer Robots (2018) www.stopkillerrobots.org/.
Department of Communications, Energy and Natural Resources (2013) National Digital Strategy for Ireland, Phase 1 – Digital Engagement, Doing more with Digital. Available at: www.dccae.gov.ie/en-ie/communications/publications/Documents/63/National%20Digital%20Strategy%20July%202013%20compressed.pdf.
European Commission (2017) Success stories about digitisation, employability and inclusiveness: The role of Europe. Available at: https://ec.europa.eu/digital-single-market/en/news/success-stories-about-digitisation-employability-and-inclusiveness-role-europe.
European Commission (n.d.) Digital Europe: Draft Orientations for the preparation of the work programme(s) 2021–2022.
High-Level Expert Group on Artificial Intelligence (HLEG on AI) (2019a) A Definition of AI: Main Capabilities and Disciplines. European Commission.
High-Level Expert Group on Artificial Intelligence (HLEG on AI) (2019b) Ethics Guidelines for Trustworthy AI. European Commission.
Möhlmann, M. and Henfridsson, O. (2019) ‘What people hate about being managed by algorithms, according to a study of Uber drivers’, Harvard Business Review. Available at: https://hbr.org/2019/08/what-people-hate-about-being-managed-by-algorithms-according-to-a-study-of-uber-drivers.
Office of the Chief Information Officer and Microsoft Ireland (2018) Enabling Digital Ireland. Available at: https://pulse.microsoft.com/uploads/prod/2018/08/Enabling-Digital-Ireland-Whitepaper.pdf.
O’Neil, C. (2016) Weapons of Math Destruction: How Big Data Increases Inequality and Threatens Democracy. Crown Books.
Hakli, R. and Seibt, J. (2017) Sociality and Normativity for Robots: Philosophical Inquiries into Human–Robot Interactions. Springer.
University of Helsinki and Reaktor (n.d.) Elements of AI. Available at: www.elementsofai.com/.
Copyright © Education Matters ® | Website Design by Artvaark Design